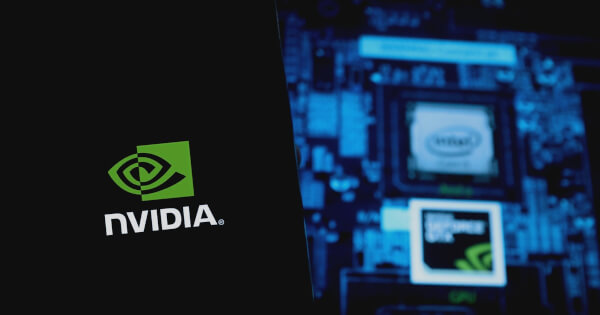
NVIDIA and Meta’s PyTorch Group Improve Federated Studying for Cell Units
Joerg Hiller
Apr 11, 2025 23:56
NVIDIA and Meta’s PyTorch workforce introduce federated studying to cell units via NVIDIA FLARE and ExecuTorch. This collaboration ensures privacy-preserving AI mannequin coaching throughout distributed units.
NVIDIA and the PyTorch workforce at Meta have introduced a pivotal collaboration that introduces federated studying (FL) capabilities to cell units. This growth leverages the mixing of NVIDIA FLARE and ExecuTorch, as detailed by NVIDIA’s official weblog publish.
Developments in Federated Studying
NVIDIA FLARE, an open-source SDK, permits researchers to adapt machine studying workflows to a federated paradigm, making certain safe, privacy-preserving collaborations. ExecuTorch, a part of the PyTorch Edge ecosystem, permits for on-device inference and coaching on cell and edge units. Collectively, these applied sciences empower cell units with FL capabilities whereas sustaining consumer knowledge privateness.
Key Options and Advantages
The combination facilitates cross-device federated studying, leveraging a hierarchical FL structure to handle large-scale deployments effectively. This structure helps thousands and thousands of units, making certain scalable and dependable mannequin coaching whereas protecting knowledge localized. The collaboration goals to democratize edge AI coaching, abstracting machine complexity and streamlining prototyping.
Challenges and Options
Federated studying on edge units faces challenges like restricted computation capability and various working techniques. NVIDIA FLARE addresses these with a hierarchical communication mechanism and streamlined cross-platform deployment through ExecuTorch. This ensures environment friendly mannequin updates and aggregation throughout distributed units.
Hierarchical FL System
The hierarchical FL system entails a tree-structured structure the place servers orchestrate duties, aggregators route duties, and leaf nodes work together with units. This construction optimizes workload distribution and helps superior FL algorithms, making certain environment friendly connectivity and knowledge privateness.
Sensible Functions
Potential functions embody predictive textual content, speech recognition, good house automation, and autonomous driving. By leveraging on a regular basis knowledge generated at edge units, the collaboration permits strong AI mannequin coaching regardless of connectivity challenges and knowledge heterogeneity.
Conclusion
This initiative marks a big step in democratizing federated studying for cell functions, with NVIDIA and Meta’s PyTorch workforce main the way in which. It opens new prospects for privacy-preserving, decentralized AI growth on the edge, making large-scale cell federated studying sensible and accessible.
Additional insights and technical particulars could be discovered on the NVIDIA weblog.
Picture supply: Shutterstock